In the UK, the government sees the deployment of AI as an opportunity to foster economic growth, and improve the efficiency of public services.
For example, the recent Spring Statement announced a £3.25 billion Transformation Fund to "support the fundamental reform of public services” and “seize the opportunities of digital technology and AI”.
This underlines the need for robust assessments of the potential risks and rewards of AI deployment in the delivery of services (“AI interventions”). Making a case for AI interventions starts with a robust business case including the future costs, benefits and risks of each investment option. The economic gains from AI adoption could be substantial. The IMF estimates that embracing AI could boost productivity by 1.5 percentage points annually. This could be worth up to £47bn to the UK each year.
Figure 1 UK GVA per hour worked (1997 – 2033)
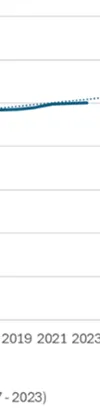
Source: DSIT and Frontier calculations
Challenges in appraising AI interventions
- AI interventions can have broad and unpredictable outcomes. They may be implemented with other changes in complex systems. There is limited evidence from past implementation of AI that we can use to quantify likely outcomes. For example, a large language model (LLM) deployed by a Government department as a citizen-facing tool may also help civil servants answer internal queries.
- AI interventions may have different impacts on different population groups because of limitations in the data used to develop the models. For example, employing image recognition to verify users’ identities may work less well for certain ethnicities.
- Making a case for an AI intervention requires comparing the intervention against a “do nothing” option. Understanding the “do nothing” option can be challenging when AI replaces or enhances complex, subjective processes. For example, if an LLM is used to help workers summarise a large number of documents, the quality of the summaries unaided by AI may be unknown.
Overcoming the challenges of appraising AI interventions
- Known outcomes, which exist currently or can be predicted with high confidence;
- Known unknowns, which can be identified but are subject to high uncertainty; and
- Unknown outcomes, which are not possible to predict with any level of confidence.
- Known outcomes could be modelled via the monetisation of non-market outcomes such as changes in wellbeing, health and the environment resulting from the intervention.
- Known unknowns require approaches that take into account greater uncertainty, such as “what-if” scenarios or Monte Carlo modelling, and some parameters may rely on expert judgement.
- Unknown outcomes are clearly the most difficult to model. Appraisers could provide a qualitative description of how the AI intervention opens up the potential for as yet undefined benefits as part of the ToC. They could also refer to the unanticipated benefits generated by similar investments.