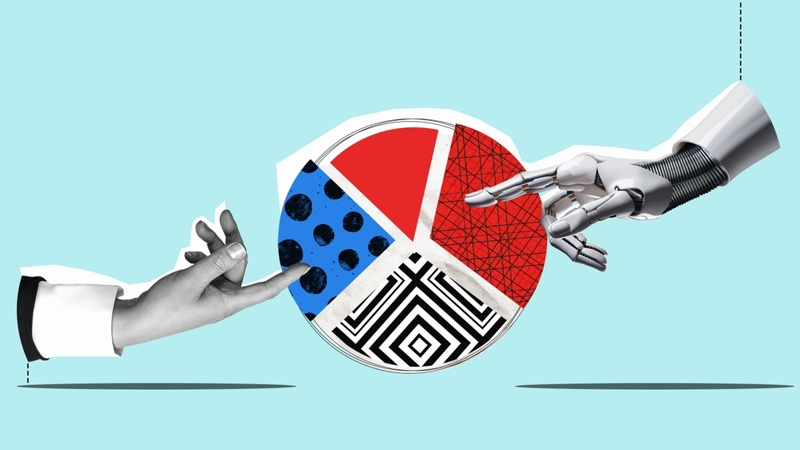
In the ever-evolving world of AI, new specialised skills are becoming essential to the UK economy. Can we use the power of AI to uncover these emerging AI skills and reshape the talent pipeline to meet the demand? Our innovative approach explores this possibility.
The elusive AI skills gap
Artificial intelligence (AI) is reshaping industries, economies, and the future job market. The UK government has been vocal about its ambition to position the country as a global leader in AI. However, achieving this goal depends on the availability of a highly skilled workforce that will enable the AI sector and the wider economy to unlock the huge potential benefits of AI.
A key challenge for sustained growth is creating a labour market with AI skills that match what businesses actually need. This could be achieved through specialised education, targeted training or focused immigration. Yet, despite sustained backing for AI development and ever-increasing investment from the private and public sectors, employers consistently report difficulties in hiring for AI roles. While this ‘demand’ vs ‘supply’ discrepancy is widely considered a constraint on transformational AI-led growth, the extent and nature of the AI-specific skills gaps are unclear. This uncertainty is reflected in the Department for Science, Innovation &Technology’s (DSIT) AI Opportunities Action Plan, which states that as a priority, the ‘government should accurately assess the size of the skills gap [in AI]’.
Traditional labour market research approaches may not be optimal for identifying gaps in AI skills. These often rely on surveys and structured interviews to gather insights, which are slow, expensive and increasingly challenging to administer. However, the hyper-fast pace of AI innovation means that many skills needed to support AI adoption are rapidly evolving in terms of their technical focus and overall scale of demand. New approaches, such as assessing vacancy data, have been deployed to address these challenges in recent years. We need to continue exploring these more innovative approaches to help policymakers identify AI skills gaps more effectively.
Frontier Economics, in partnership with Glass.AI, sought to test a new method to explore the current demand for AI skills in the UK labour market and whether university graduates are likely to meet this demand. Using AI tools, we analysed the skills gaps that emerged when data on the AI skills employers require in their job advertisements was compared with those taught in UK university courses.
Our proof-of-concept method is agile, scalable, and repeatable – offering a fresh perspective on one of the labour markets’ most pressing research challenges.
Our approach to assessing the AI skills gap
To understand the AI skills landscape, we analysed two publicly available data sources:
- AI skills demand: job advertisements from LinkedIn represent the vacancies and skills employers seek.
- AI skills supply: university course descriptions (24 Russell Group institutions only) representing the skills universities teach.
Glass.AI harnessed the power of its AI research capability to obtain skills supply and demand data from the open web. Glass.AI’s technologies enable deep reading of global web content that assesses sectors and companies’ characteristics by applying AI in a specific context – machine language understanding.
Using a targeted crawling approach and by deep reading LinkedIn and a select subset of university websites from the Russell Group, Glass.AI gathered:
- 1,440 job adverts
- 3,573 course and module descriptions
The crawl was directed by linguistic cues, including phrases and descriptions that would support the discovery of relevant data.
Next, Frontier’s data science team analysed this rich data that was comprised of unstructured text. We used large language models (LLMs) to extract 7,639 unique in-demand techniques and skills from the job adverts and mapped these into 21 key groups, which we called ‘skill domains’.
We then applied natural language processing (NLP) techniques to analyse the skill domains, uncovering key gaps between what is being taught and what businesses seek. This delves into the core of labour market dynamics and takes insights beyond what can be achieved using conventional research methods.
What are the gaps in the AI talent pool
Our exploratory analysis highlights several trends that might be important to the UK’s AI talent market:
1. A shortage of some key AI skills with university courses not obviously filling the gap in the short term
The data suggests a potential under-supply of graduates with expertise in two in-demand AI skill domains: NLP and Cloud Computing. Our innovative approach allows us to dig into these gaps and understand what could be causing them.
- NLP: The surge in demand for NLP skill domain, such as prompt engineering, is almost certainly linked to the rapid rise of generative AI, which has transformed how businesses think about automation and human-machine interaction.
- Cloud computing: While there is a clear gap between demand and supply, our analysis shows that cloud computing skills are more frequently required in more senior AI roles. There is a substantial increase in importance between associates and mid-level positions, which may suggest that cloud computing is typically learned on the job. However, given the centrality of cloud platforms in developing and deploying AI models, policymakers may want to increase their focus on building cloud engineering skills in the UK workforce.
2. A possible priority mismatch
Some skills seem widely taught at universities but are rarely mentioned in job descriptions. This could reflect one of two dynamics.
· Employers may assume that specific foundational AI skill domains, such as statistical analysis, are implicit in candidates’ backgrounds and do not list them in their job advertisements.
· Universities may overly focus on areas that do not align with immediate industry needs, creating a potential disconnect in graduate employability.
What does this mean for policy and businesses
These results are indicative and based on a large, yet limited, ’proof-of-concept’ sample. However, our approach could be easily scaled to cover a broader range of education provisions and job postings from other sources.
Although our findings are preliminary, they point to three interventions which may help:
- Real-time skills monitoring: The traditional approach to labour market research is too slow for the fast-paced AI industry. AI-driven methodologies, like the one we have developed, offer a repeatable and scalable way to track demand shifts in real time and support more agile decision-making.
- Updates to university curricula: Universities and other providers of further and higher education may wish to consider expanding AI curricula to incorporate skill domain demands such as cloud computing and NLP. The government could explore whether any barriers prevent a more rapid update of curricula and offer policy levers to assist universities in overcoming these challenges.
- Immigration policy: In the short term, attracting high-skilled AI professionals in areas with the most acute gaps from abroad could help bridge the gap while domestic training systems adjust.
In addition, our results show that businesses also have a role to play through:
- Better communication with universities: Employers need to clearly articulate their AI skills requirements, ensuring that universities and other training providers can align their programmes with market demand.
- Investment in on-the-job training: Some in-demand skill domains, such as cloud computing, may be learned in the workplace. Companies could consider building structured training programmes to accelerate workforce upskilling. In more junior roles, these training programmes will bridge education gaps, and in more senior roles, they can replace formal education.
- Anticipating future skills needs: AI adoption is increasing across industries. Companies that take a proactive approach to AI talent development and engage closely with labour market trends will have a competitive advantage.
What are the advantages of our innovative methodology
Our noval approach to assessing AI skills gap offers several important advantages:
· Agility: Advanced AI research technologies (machine language understanding and generative AI models) can automate data collection and the analysis of job adverts and course descriptions, making skills mapping much faster than traditional survey-based methods.
· Scalability: Our approach is not limited to AI. It can be effectively applied in other high-priority sectors such as green energy, cybersecurity and the creative industry, offering valuable insights into workforce trends and the labour market pinch points, which may be limiting growth.
· Repeatability: This methodology can be replicated frequently and consistently, allowing real-time tracking of skills shortages as the AI labour market evolves.
Looking ahead
AI is an ever-growing influence in our lives and is shaping the future labour market. Ensuring that the UK has the right talent to support its ambitions of being a global leader in AI remains a fundamental challenge. We need an accurate, real-time view of the existing skills gaps to address this challenge effectively and efficiently.
What better way to blaze a trail than by harnessing the powerful AI tools to understand labour market challenges? Using AI technologies to analyse skills gaps, we can track workforce trends, inform policymakers, support policy decisions, and ultimately help shape the future of AI talent in the UK. In a new age of policymaking challenges, AI approaches provide agile, scalable and repeatable solutions.