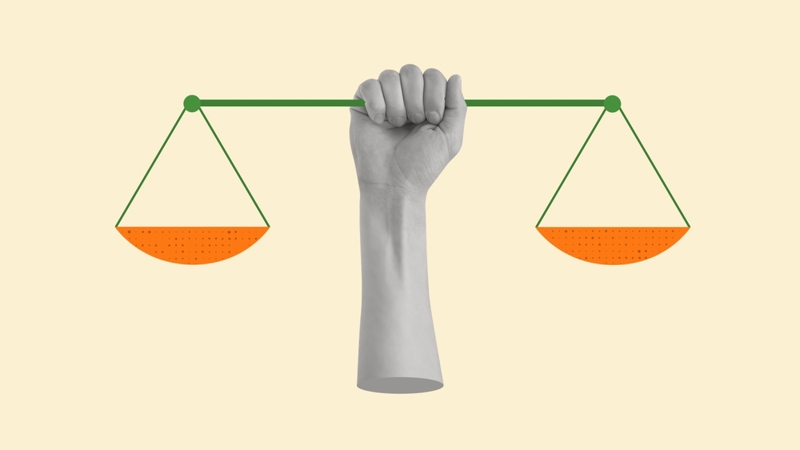
In recent years, regulators have intensified their scrutiny of the practices of digital platforms, (e.g. the GAMMANs – Google, Amazon, Meta, Microsoft, Apple, and Netflix).
New laws, such as the UK's Digital Markets, Competition and Consumers Act (DMCCA) and the EU's Digital Markets Act (DMA), have given authorities additional powers to address potential anti-competitive behaviours. Alongside this, there has also been a rise in class action lawsuits against digital firms in the UK (sometimes building on precedent from regulatory cases)., as well as disputes over contractual obligations related to algorithms and its usage, particularly in digital assets and digital finance. As algorithms became a mainstay in the markets, both litigation and arbitration cases in these areas are only expected to rise.
Algorithms, often proprietary and data-driven, are core to the operations of all digital operations, impacting how consumers engage with their services. An assessment of whether algorithm-driven behaviours are anti-competitive or constitute a breach of contract pose significant hurdles and require innovative approaches and a multidisciplinary skill set.
Why can algorithmic decision-making raise concerns?
Concerns surrounding anti-competitive conducts
Algorithms are step-by-step instructions that help computers process large amounts of data, make complex calculations or automate tasks. Advances in machine learning and access to vast datasets have enhanced algorithmic capabilities, allowing businesses to identify patterns, make predictions, and make decisions and recommendations autonomously, as observed in the use of dynamic pricing in ride-hailing, sports ticketing, and hotel booking services. However, as algorithms become more advanced and firms collect more data on their customers, algorithmic decision-making may raise competition concerns or raise questions on contractual relationships. Legal experts and arbitrators will need to assess how algorithmic practices affect competition, and whether there are potential breaches of contract in how the algorithms are designed, developed and implemented.
Several recent cases highlight these issues from the perspective of anti-competitive effects:
■ In the seminal case of Google Shopping, the European Commission found that Google had given preferential placement to its own comparison-shopping service, while demoting those of its competitors in its search platform – Google Search. Google argued that its algorithm penalised comparison-shopping services in order to prevent poor quality sites from appearing in its search results, but the EC found that the algorithm applied different standards to Google’s own service.
■ Amazon is another firm that was under regulatory scrutiny (e.g. from the CMA, EC and the AGCM), which might also be facing a class action claim in the UK. Amazon uses an algorithm to determine which seller is featured in the Buy Box – the section of the product detail page that consumers interact with to add products to cart or buy immediately. Several competition authorities found that Amazon Retail was disproportionately more likely to be featured in the Buy Box when competing with third-party sellers, raising questions about whether the algorithm systematically favoured Amazon’s own retail arm.
■ In the Trivago case, Australian regulators found that the company’s hotel price comparison algorithm placed significant weight on commissions paid by hotel booking sites rather than offering the lowest price to consumers, as purported by Trivago.
Concerns surrounding contractual breaches
There are also cases relating to (potential) contractual breaches. A large number of cases brought to the Business and Property court in the UK were related to digital assets and digital finance. These cases delved into a variety of questions regarding contractual obligations related to algorithms For example, there was a termination dispute in relation to an IT system which required understanding whether the source code (i.e. the algorithm) had been developed, copied or re-written. Another dispute concerned the closure of an online trading account used in trading futures with the issue being whether the platform provider was entitled to do so and the relief which should follow if it was not. These are just two cases among many. Potential for contract breaches may likely increase, as more products and services – from ride-hailing (e.g. Uber) to streaming services (e.g. Netflix) – include binding arbitration clauses. In such cases, arbitrators would have to not only review contractual obligations but be able to unwind the algorithmic decision-making to understand the implications.
How to assess algorithmic decision-making for anti-competitive behaviour or contractual breaches?
In competition and arbitration disputes, assessing the impact of algorithmic decision-making requires a rigorous multi-disciplinary approach involving both technical and economic aspects. Two key issues arise in this context: (a) Identifying the appropriate tools for engaging with the algorithm, and (b) Identifying the relevant counterfactual.
Identifying the appropriate tools for engaging with the algorithm
In disputes involving algorithmic decision-making, the choice of analytical tools depends on the access to the algorithm, through code and technical documentation, and the data used to train it. These approaches can be broadly categorised into three categories:
■ Governance audit: This includes a range of techniques to review the documentation of an algorithmic system and the processes used to develop and monitor it. Technology specialists and data scientists at the CMA used this approach when investigating Meta’s alleged use of advertising data from advertising customers to advantage its development of Facebook Marketplace.
■ Empirical audit: If reviewing code alone is not conclusive – for example, as outcomes depend on how the algorithm interacts with users and the market – an empirical investigation can be carried out with data sourced directly from the organisation or obtained through other means such as web-scraping. In the case of Google Shopping, the EC analysed 1.7 billion search queries and simulated changes in ranking to observe differences in user engagement.
■ Technical audit: These methods involve opening up the algorithmic system to understand how the algorithm(s) works and interact with other systems and processes, and is the most comprehensive of the three. Methods include reviews of computer code and technical documentation and adapting or simulating the algorithm so that it can be re-run. Data scientists used this approach to Trivago’s ranking algorithm, including reviewing the underlying code and re-running the algorithm using test data, in the ACCC v Trivago case.
However, even if access to the algorithm is unavailable, alternative methodologies – such as econometric analysis and indirect inference techniques – can still meaningfully assess algorithmic outcomes. Researchers are developing methodologies that do not require direct access to an algorithm but instead isolate its effects from external factors – for example, some researchers devised an approach to distinguish personalisation effects from background noise, assessing the role of variables such as operating system choice and purchase history in the context of price-discrimination on e-commerce platforms.
Identifying the counterfactual
Any cases involving potential anti-competitive conduct or contractual breaches require deriving a suitable counterfactual, which can be based on the algorithm itself, or rely more heavily on economic theory.
Counterfactuals based on the algorithm itself ensure greater accuracy in assessing competitive effects or potential contractual breaches. For example, an audit may highlight manual adjustments or assumptions within the code that generated the disputed conduct. A counterfactual can thus be generated by removing such assumptions from the code itself. In the carriage disputes on potential class action cases against Amazon, the Competition Appeal Tribunal’s (CAT) favoured this approach of removing alleged anti-competitive conduct from Amazon’s algorithm itself. The South African Competition Commission (SACC) also required algorithm-based remedies from Meta – one of the remedies involved changing the Facebook algorithm or reversing algorithmic changes that were designed to deprecate SA news content on Meta.
Constructing a reliable counterfactual while removing potentially problematic elements from an algorithm is not without difficulties, as adjustments to one component can have unintended ripple effects. For example, removing one factor from an algorithm may not only alter immediate outcomes but also influence how the algorithm adapts over time. Moreover, even when algorithmic adjustments have been proposed as a remedy, regulators and courts are often not prescriptive – for example, in the Amazon Buy Box cases, neither the European Commission nor the CMA’s commitments outlined a precise methodology for ensuring non-discrimination. However, the appointment of an independent monitoring trustee (whose selection is vetted by the regulators) at least ensures that the companies remain compliant with the algorithmic adjustments they proposed.
Econometric techniques used in more traditional competition cases can be used to construct a suitable counterfactual and assess the quantum of damages, especially when access to the algorithm is limited. In more traditional cases, such as cartel investigations, econometric models help assess whether prices might have differed absent the alleged conduct and by how much. These models can be adapted to evaluate algorithmic decision-making, absent the disputed conduct, using available data. For example, researchers have investigated self-preferencing in Amazon’s Buy Box selection, search rankings, and on items frequently bought together. These studies did not have access to the Amazon algorithm, and some, did not have access to Amazon’s proprietary data either. Despite these challenges, the studies where able to identify if the way the algorithm is designed led to self-preferencing of Amazon Retail products in Amazon’s marketplace.
Some tools to assess the algorithm, e.g. the technical audit, can also complement the construction of an econometric model. By building a solid understanding of the data and methods used in the algorithmic system, the expert can design an econometric framework that includes the most important drivers of the outcome of the algorithmic system, and that reflects the way in which the algorithm weighs these drivers. This minimises the econometric model's ‘error term’, i.e. the unexplained variance in the outcome of interest, allowing for a more robust analysis.
Assessment process for arbitration
The process of an arbitration to review potential contractual breaches in algorithmic conduct can be simplified into two broad categories: (a) an assessment of merits, and (b) an assessment of the quantum.
In merits-based assessments, arbitrators may examine, for example, whether the algorithm's design and implementation align with the terms agreed upon in the contract or, if the use of the algorithm adheres to implied duties of fair dealing and good faith (often a part of commercial contracts). Arbitrators may also consider whether the contract appropriately allocates the risks of algorithmic errors or unintended outcomes to the responsible party.
In assessing quantum, arbitrators must establish causation – demonstrating that the claimed losses resulted directly from a contractual breach involving the algorithm’s design or implementation. They must also calculate the financial losses suffered as a result of the algorithm’s failure to perform as contractually promised.
To assess both merits and quantum, arbitrators will rely on the same tools outlined earlier, which are used by both competition economists and data scientists – engaging with the algorithm through, for example, an audit to identify potential breaches and constructing an appropriate counterfactual by re-running the algorithm itself or via simulation or econometrics to estimate the financial impact.
Way forward
Assessing algorithmic decision-making requires both economic and technical expertise. Just as in more traditional competition and damages cases, determining the appropriate but-for world requires a solid understanding of economic principles to establish the most relevant theory of harm. Choosing the relevant counterfactual relies heavily on understanding the case and identifying the applicable theory of harm.
At the same time, engaging meaningfully with algorithmic decision-making requires data science expertise. This is critical not only for analysing the algorithm’s design but also for adjusting it or developing relevant simulation exercises to test the impact of the alleged conduct. Without this interdisciplinary approach, the risk of misinterpreting the effects of an algorithm – and, consequently, drawing incorrect conclusions about competitive harm – remains high.
Frontier Economics is a recognised industry leaders in dispute support, working on antitrust and competition damages, investor-state disputes, and commercial disputes. With an expertise in economics and data science, Frontier Economics is uniquely placed to support in cases involving algorithmic conduct, as demonstrated in the recent carriage dispute on Stephan v Bira (v Amazon), where the CAT noted, “Altogether, we found that Dr. Houpis’ comprehensive report presented an impressively well-developed and thought through methodology.”